02 Jun Algorithms and Image Biomarkers. Radiomics as another piece of precision oncology
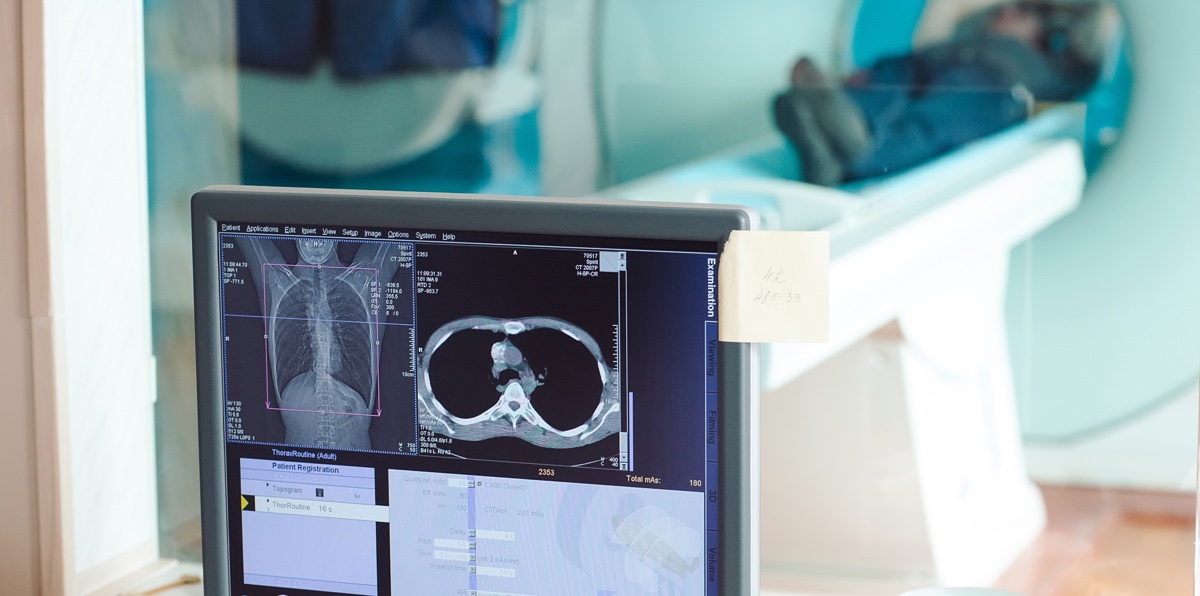
Radiomics is revolutionising medical image interpretation.
Cancer is a disease with a high incidence rate among the population (442.4 cases per 100,000 people). And its mortality rate stands at 158.3 per 100,000 people. By 2040, the number of new cancer cases per year is expected to rise to 29.5 million people and the number of cancer-related deaths to exceed 16.4 million.
Medical images such as computed tomography (CT scan) or magnetic resonance imaging (MRI) play a fundamental role in the detection, diagnosis and follow-up of cancer in clinical practice. However, they are not sufficiently accurate in cancer screening. Digital imaging data can be calculated using mathematical algorithms that allow the extraction of a substantial amount of information about tissue intensity, shape, and texture, providing an excellent tool to improve the image data interpretation and biomarker development.
Radiomics
The application of advanced computational analysis to image data opens a new paradigm in the field of radiology. Radiomics is revolutionising the evaluation of medical images and their interpretation, moving from a subjective evaluation to a quantifiable one. The image, being made up of small pixels, hides information that can go unnoticed by the human eye and, by applying these algorithms, it is possible to extract and quantify relevant data on tumours from medical images. Based on these data, and after exhaustive validation, algorithms can be established not only to diagnose a tumour earlier but also to know if the tumour is more or less aggressive, and even predict the response to certain treatments.
This will allow physicians to have tools to monitor patients undergoing treatment and make faster decisions.
A current workflow includes the following stages: acquisition of the images, incorporation of these into the patient’s history and exporting the set digitally to the Radiomic software. At the end of this process, a mathematical model is generated that will be able to predict the parameter of interest and that will be validated with the rest of the clinical data.
Artificial intelligence and machine learning can be helpful in radiomic evaluation. Also, neural networks will be able to perform massive texture analysis and training of the data to create prediction algorithms.
From GENESIS Biomed we are convinced that the development of radiomics will introduce changes in the clinical practice, which will translate into a more effective precision oncology with improvements in the mortality rate and, therefore, in an increase in cancer patient survival.